AI and environmental challenges
The growth of artificial intelligence is impossible to ignore, but how does it intersect with climate and the environment? Law professor Cary Coglianese and engineering professor Benjamin Lee weigh in on the roles AI may play.
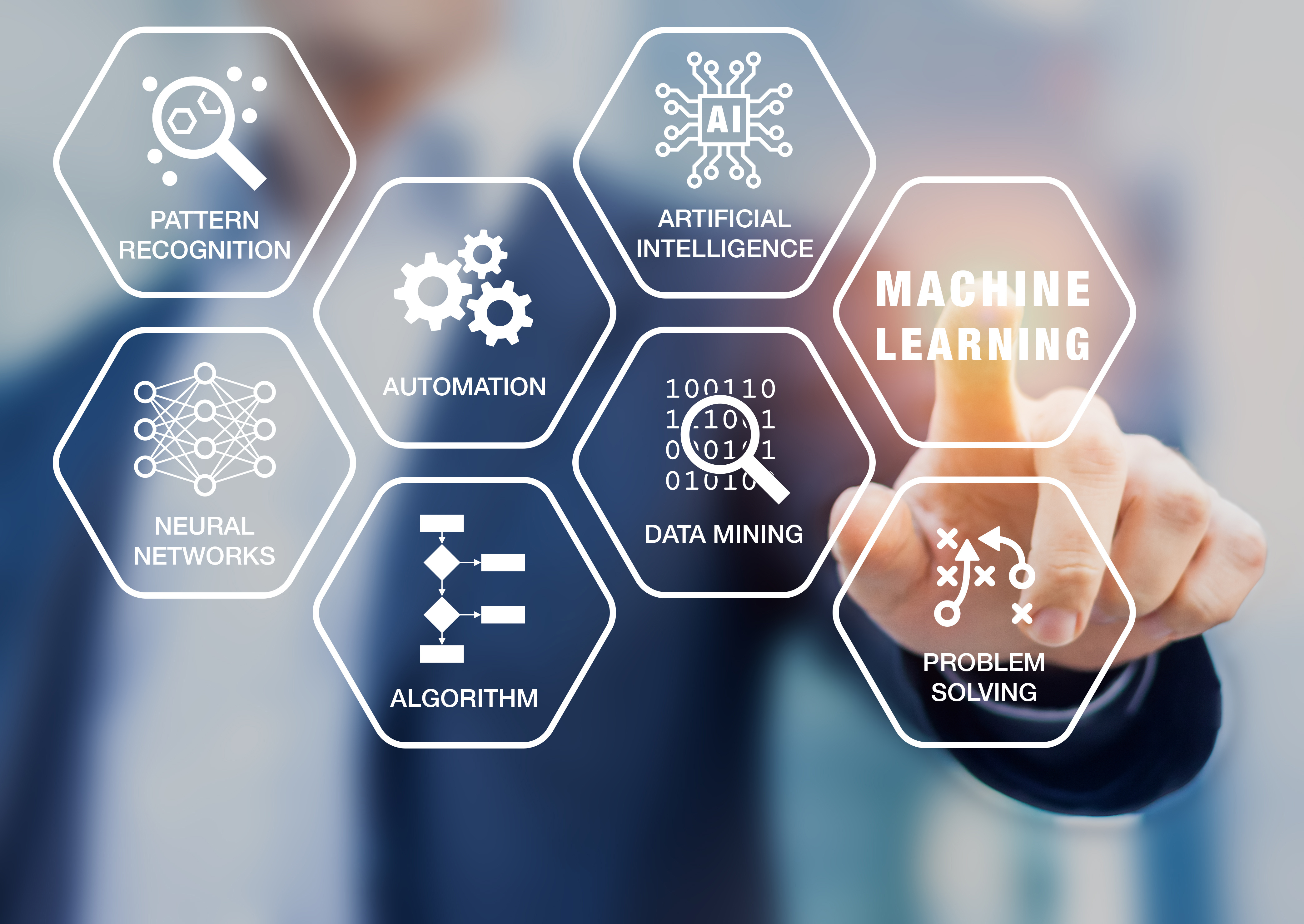
By Molly Flanagan
Artificial intelligence (AI) is now inescapable, a subject discussed around the globe. AI’s growth has been met with both excitement and concern across several industries. Yet AI also has a role to play in the environmental world. The technology has the potential, for example, to solve complex problems in the context of combating global climate change. AI can also expedite technological advances, speed the development of new and more sustainable materials, improve energy efficiency, inform disaster response, and forecast environmental processes like erosion, landslides, and weather patterns.
These advances do not come without risk. AI may contribute to job displacement across several industries, exacerbate resource and energy use, and increase the circulation of misinformation and bias. For these reasons, it is essential that AI is regulated in an ethical and responsible manner to ensure the safety and protection of public interest.
We asked two of Penn’s esteemed faculty members, Cary Coglianese, professor of Law and Political Science, and Benjamin C. Lee, professor of Electrical and Systems Engineering and Computer and Information Science, to use their distinct expertise and disciplinary backgrounds to shed light on the impact of AI on environmental challenges in this Q&A.
First of all, what is artificial intelligence?
Lee: Artificial intelligence is the idea that you can take tasks that were originally designed or done by humans and program a computer to do them, hence the term “artificial.” Artificial intelligence has been studied for a long time, but we saw a major breakthrough in the 2000s due to the massive amounts of data available to teach computer programs how to make connections and perform tasks. This process is referred to as machine learning.
Coglianese: There are many understandings of what counts as artificial intelligence. For instance, my thermostat that adjusts automatically after I program it might be thought of as artificial intelligence. But I generally think of it as the application of machine-learning algorithms to predict outcomes to complex problems and to identify patterns. AI can be built into everything from financial management systems to online troubleshooting chatbots. They can act as a system to support, or even at times replace, human decision making.
How does AI relate to climate change and the environment?
Coglianese: We’re entering an era where we have to optimize our natural resources, such as water, clean air, fertile soil, and precious metals. There are all sorts of resource scarcity and optimization problems where artificial intelligence can really help us.
Another application of AI that I think is vital going forward in dealing with the climate crisis is for electricity grid management. When you have many smaller, distributed sources of electricity production, such as solar panels on people’s roofs, rather than a larger, concentrated source, such as a coal-fired power plant, AI can help manage the complex electric grid. By accurately forecasting supply and demand for energy, AI can offer electricity optimization strategies, which will become more and more important in the overall transition to renewable energy sources.
I could go on with a number of examples where AI prediction forecasting is important for issues such as flood management, storm responses, and reducing global carbon emissions. AI can generate complex forecasts to model future scenarios in real-time. All sorts of climate resilience and adaptation work would benefit from artificial intelligence as well. AI can also be used for complex analysis and progress tracking of pollution prevention or waste reduction goals, such as those related to air quality or corporate carbon footprints.
Lee: I think a lot about energy used by AI. Looking at massive high-performance data centers, like the ones built by Google or Amazon, the question is: how much energy is being used to power these data centers, and how rapidly is that number growing? Researchers have found that as a percentage of total energy, information technology accounts for only 1% globally and has not changed over the past few years. 1% is big, but it’s not huge, and people are surprised to learn that the growth in energy usage has not been much larger. Big technology companies have achieved economies of scale with their data centers, which has improved energy efficiency and kept costs low for data center computing. At the same time, AI is certainly driving a lot of new data center construction at, for example, Microsoft. I would be surprised if, five to ten years from now, we are still at 1% of total energy. Much of that growth will be driven by AI.
Generative AI models, like ChatGPT, are going to be much more computationally expensive than traditional data center jobs. For instance, if a Google web search consumes some amount of energy, asking ChatGPT to give you an answer might consume 7 to 10 times more energy. If everyone starts using GPT like they use the search engine, we will see a big increase in energy costs. Beyond today’s search engines, we expect many new applications will be enabled for the first time with generative AI.
How can AI grow in a sustainable way? What are some barriers involved?
Lee: There was a lot of talk about sustainability two to three years ago in the technology industry, and companies are continuing to set ambitious goals for sustainability. However, the advent of AI has changed the conversation and shifted priorities in a significant way. Companies have begun taking an “all hands on deck” approach to position themselves best for developing and deploying AI in production applications and systems.
There needs to be a big step up in the amount of renewables installed. The Inflation Reduction Act might lead to a bigger increase in investments in renewables that could help. There is a hope that AI usage could become carbon neutral if it’s powered by renewable energy sources, but I think we are far away from that. Increasingly, we think that batteries will have a big role to play in energy storage, which could support the broader transition to renewables.
Coglianese: Moving AI forward so that it relies on a more sustainable source of energy is going to go hand in glove with the transition of the overall economy’s transition to sources of energy that are renewable—that is, not oil, gas, or coal. The research into how to make this transition happen is ongoing, but looking for more efficient sources of energy for manufacturing, production, and transportation is certainly an important area to explore. I think the main policy issue with AI today is how to manage the fast pace of innovation, especially in the private sector, while also protecting the public from some of the risks associated with artificial intelligence, such as privacy, bias, and manipulation.
How can AI impact environmental policy and regulation?
Coglianese: AI has been useful in identifying potential violators of environmental regulations. There are hundreds of thousands of permitted, EPA-regulated facilities, such as heavy industrial sites or fossil fuel power plants, around the United States. That’s far more than the number of facilities that government inspectors can inspect or evaluate for compliance on a regular basis. So, we have to figure out how to best allocate our sparse inspection resources amidst this very large pool of potential violators. Some of my research suggests AI can be remarkably effective at forecasting which facilities are likely to be violating environmental regulations; we should go and get those facilities inspected, and that way be much more efficient with our limited resources. Another group’s study showed that, for example, in comparison to just a random selection of facilities, the EPA could improve its detection of water pollution violators by over 600% by using machine learning algorithms. That is just one example where this technology can be useful.
Beyond supporting environmental compliance, AI can be used in satellite monitoring to track global climate change impacts and progress on sustainability targets on several scales.
Knowing that AI can develop bias, how can this be mitigated, especially in the context of environmental justice?
Coglianese: It’s important to start by noting that AI gets its power from the data on which its algorithms train. Much of the data that exists today has been human-generated, and humans have biases that have crept into that data. So the risk is, if AI is trained without much care or thought on data that have biases built into them, then the outcomes will simply reflect those biases. I think that, speaking generally, AI has the potential to create more fair, accurate, and efficient systems across a whole range of applications when you compare it with human decision making that has implicit biases. This isn’t to diminish the serious concerns that exist about bias being replicated in AI algorithms, especially if they’re not handled in a thoughtful and responsible manner. It is to offer some possibility that we might even be able to do better with some of these digital tools in some circumstances, if we use them with care.
How do we go about regulating AI?
Coglianese: Across the board, there’s not a “one size fits all” type of action that everyone needs to take to make sure their AI doesn’t cause problems. What amounts to a fair, safe, transparent, accountable algorithm in a pacemaker would be different from what is fair, safe, and suitably transparent in a self-driving car. And both of those would be different than an algorithm driving a social media platform. I have argued that we need to think about regulating artificial intelligence in a way that we’ve used in other contexts where there is a great deal of heterogeneity. This approach is called management-based regulation; instead of telling companies exactly what to do, what we can tell them is how to approach and manage their own AI development and deployment processes. The government just needs to know what a good impact assessment or auditing process looks like for AI algorithms. I think that’s the direction we are likely to go, and bias would be one of the things that a responsible AI impact assessment or auditing process should look for.
Is there anything you would like to share that gives you hope?
Lee: What gives me hope is that there are so many people thinking and talking about AI and sustainability, both in industry and academia. I think the solution space is well known, it is just a matter of optimizing the solution and striking the right balance. We know roughly the contours of what needs to happen, like how we talked about renewable storage and job scheduling, which allows renewable energy to be stored until it is needed later. Beyond that, computer scientists need to figure out where in this big solution space we want to be, and whether we can implement the solution effectively. I think there are many ways to get to more sustainable computing.
GPT promises massive increases in productivity. It’s a tool that will transform the way we live and work, so I do think that AI should be given a little bit of room to run before we say we need to bring in carbon neutrality to AI computing. AI is still in its early stages of development, relatively speaking, and I think it will certainly become more efficient than what we’ve got today through continuing advances in both software and hardware.
Coglianese: We’re entering an era where we have to do better, and more, with less. If AI can be deployed responsibly, it can be used to help in solving a host of society’s problems and concerns; it is being used increasingly in both the private sector and governmental programs that aim to have it be a force for public good. What gives me hope and optimism for AI, then, is its potentially greater capacity to help solve complex policy problems that are becoming exceedingly difficult. Technology won’t be the only answer, but it is important to improve the information basis for decision making.
But to use AI well, and to regulate it well, also requires that we as humans recognize that regulatory excellence is often “people excellence.” In other words, we need people to use these AI tools wisely and well, since they won’t work on their own to promote public good. The usefulness of AI requires a creative spark from real people to identify areas where this technology can be used most effectively and then to design it with care and attentiveness. Those who have raised alarms about AI and its dangers ought to be applauded, and their concerns need to be heeded. We need to proceed with management systems and auditing processes designed to monitor for problems that arise—and then fix them.
I will lastly say that one of the key things that keeps me optimistic about humans’ potential to use technology responsibly are the great people that I get to work with in the course of my research and teaching, especially my students and colleagues here at Penn. They really give me hope that we can get through difficult times and use technology appropriately to help overcome the daunting challenges that lie before us.
Experts
Cary Coglianese is the Edward B. Shils Professor of Law and a Professor of Political Science. He is the founding Director of the Penn Program on Regulation. Coglianese is a specialist in the study of administrative law and regulatory processes and has recently written on climate change policy and the use of artificial intelligence (AI) by government agencies and environmental programs; in particular, he has written Deploying Machine Learning for a Sustainable Future, and Regulating Machine Learning: The Challenge of Heterogeneity. He is an affiliate of The Warren Center for Network & Data Sciences and AI@Wharton, as well as a non-resident fellow of the Information Society Law Center at the University of Milan.
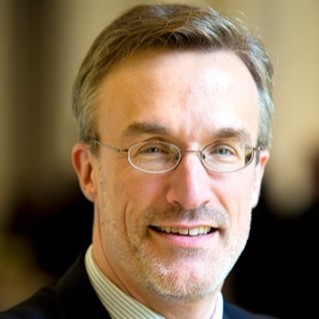
Benjamin Lee is a Professor in the Departments of Electrical and Systems Engineering, and Computer and Information Science at Penn. He is an affiliate of The Warren Center for Network & Data Sciences and was recently a Visiting Researcher at Meta AI. His research focuses on computer architecture, energy efficiency, and system security. Lee was previously featured in Penn Today, in conversation surrounding the energy and resource problems associated with artificial intelligence (AI) computing. He will be presenting at the upcoming workshop, Towards Environmentally Sustainable AI, part of the year-long AI and Climate Change series hosted by the Penn Program on Regulation.
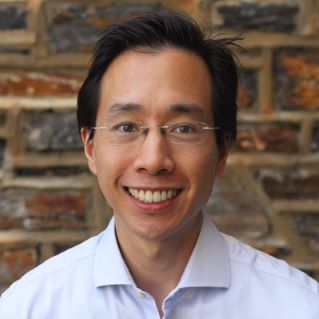
Learn more
Glossary
Algorithm: In the context of artificial intelligence, an algorithm may be understood as the “rulebook,” or a recipe, that guides a machine or program to learn, make decisions, and operate independently.
Climate change: Climate change refers to the long term variations of natural processes - like weather patterns, global temperatures, sea level shifts, etc. - that occur naturally or by humans. Since the Industrial Revolution (1800s), human activities have been the overwhelming catalyst of climate change, particularly from rising greenhouse gas emissions associated with the burning of fossil fuels.
Data center: A data center is the physical facility which houses the infrastructure required to support computing machines and equipment, such as the information technology (IT) infrastructure required for a business to run applications, services, and manage operations.
Energy efficiency: Energy efficiency is a concept that refers to the use of less energy to achieve the same output or end result. Processes that are energy efficient minimize the amount of energy that is wasted, thus reducing energy costs and carbon emissions. For instance, a home equipped with energy-efficient appliances (e.g. washing machine, refrigerator, HVAC) will have lower electricity bills as compared to a home with standard appliances. This is because the technology in the energy-efficient appliances requires less energy to achieve the same output, or complete the same function.
Environmental justice: Environmental justice is a concept that describes the fair and equitable treatment of all people regardless of race, class, national origin, gender, etc. in environmental policy planning and regulation. The Department of Energy (DOE) further defines “fair treatment,” explaining that “...no population bears a disproportionate share of negative environmental consequences resulting from industrial, municipal, and commercial operations or from the execution of federal, state, and local laws; regulations and policies.”
Environmental racism: Environmental racism is a form of systemic racism, and is defined by the disproportionate burdening of environmental and public health hazards, like air and water pollution, on communities of color. These impacts are created by policies and practices which locate industrial sources of toxic waste, such as power plants, landfills, mines, and sewage treatment operations, in close proximity to communities of color who, as a result, experience higher incidents of health impacts. The NAACP asserts race as the “...number one indicator for the placement of toxic facilities in this country.”
According to the World Economic Forum, Civil rights leader Benjamin Chavis coined the term in 1982, defining it as: “Racial discrimination in environmental policymaking, the enforcement of regulations and laws, the deliberate targeting of communities of color for toxic waste facilities, the official sanctioning of the life-threatening presence of poisons or pollutants in our communities, and the history of excluding people of color from leadership of the ecology movements.”
Fossil fuels: Fossil fuels are energy sources that are naturally derived from Earth’s geological past, hence the term “fossil.” Fossil fuels are non-renewable resources, which means their stocks are depleted as they are used. Fossil fuels contain hydrocarbons, and their combustion results in the emission of greenhouse gasses like carbon dioxide, methane, and nitrous oxide. Examples of fossil fuel sources include coal, oil, and natural gas.
Machine learning: Machine learning is a type of artificial intelligence. It refers to the ability of machines to mimic human learning and intelligent behavior through the use of data and algorithms. A machine learning algorithm is taught to recognize patterns, and make decisions and predictions, by being fed large amounts of preprocessed data. Essentially, data can be thought of as the “food” or fuel that powers machine learning. Examples of machine learning include facial recognition and personal recommendations from a streaming service, like Netflix.
Renewable energy: Renewable energy refers to energy that has been derived from a natural source that cannot be depleted, or is self-replenishing. Examples of renewable energy sources include wind, solar (sun), hydroelectric (water), and biomass power.
Sustainability: At its most basic level, sustainability may be understood as the ability to meet the various needs (social, economic, environmental, etc.) of the present without compromising or sacrificing the ability for future generations to meet their various needs. Sustainability as a concept emphasizes the interconnectedness of issues such as social equity, environmental health and wellbeing, economic vitality, and long-term resilience. Overall system sustainability depends on a balance of social, environmental, and economic sustainability factors. Specific definitions from Circular Ecology: [1]
Environmental: “Environmental sustainability means that we are living within the means of our natural resources. To live in true environmental sustainability, we need to ensure that we are consuming our natural resources, such as materials, energy fuels, land, water…etc, at a sustainable rate. Some resources are more abundant than others and therefore we need to consider material scarcity, the damage to environment from extraction of these materials, and if the resource can be kept within circular economy principles.”
Economic: “Economic sustainability requires that a business or country uses its resources efficiently and responsibly so that it can operate in a sustainable manner to consistently produce an operational profit. Without an operational profit, a business cannot sustain its activities. Without acting responsibly and using its resources efficiently, a company will not be able to sustain its activities in the long term.”
Social: “Social sustainability is the ability of society, or any social system, to persistently achieve a good social well being. Achieving social sustainability ensures that the social well being of a country, an organization, or a community can be maintained in the long term.”